Revolutionizing Artwork Classification With an AI-Powered Image Classification System

Background
The art industry faces a significant challenge in efficiently managing and categorizing artwork images. Traditional manual classification methods are time-consuming, inconsistent, and prone to human bias. These limitations hinder the ability to manage large artwork datasets, impacting collection management, research, education, and the art market. The need for an automated artwork image classification system to analyze and classify artwork images based on attributes like style, genre, type, description, materials, period, and geographic origin has become critical.
Objective
The primary objective was to develop an AI-driven image classification system capable of automatically analyzing artwork images and categorizing them across multiple attributes. This initiative aimed to address inefficiencies, improve discoverability, and enhance accessibility for art curators, researchers, artists, and art enthusiasts alike.
Approach
To tackle this problem, the team employed a structured five-phase approach:
- Project Initialization and Planning
- Defined the project scope, outlined a detailed plan, and assigned tasks.
- Data Preparation
- Acquired diverse datasets, conducted data exploration, and performed preprocessing.
- Shared an initial demo showcasing insights and progress.
- Model Development and Initial Training
- Built the first iteration of the classification model, emphasizing a robust framework for accurate categorization.
- Model Refinement and Second Evaluation
- Refined the model using hyperparameter tuning to optimize performance and reliability.
- Finalization and Deployment
- Finalized and deployed the model as an API endpoint for scalable and efficient artwork classification.
Tools and techniques included machine learning algorithms, comprehensive datasets, and iterative evaluation to ensure the artwork image classification system provided high accuracy and usability.
Results and Impact
The project successfully delivered a Minimum Viable Product (MVP) that automates artwork classification with significant improvements in accuracy and scalability. Key outcomes included:
- Enhanced Efficiency: Reduced the time and resources required for manual classification.
- Improved Discoverability: Enabled better tagging and categorization, improving access for curators, researchers, and enthusiasts.
- Increased Market Value: Enhanced accessibility and presentation positively influenced valuation and sales in the art market.
This innovation offers a scalable solution that can transform digital art curation, making it more dynamic, accurate, and user-friendly, thus enhancing the utility of an image classification system in the art industry.
Future Implications
The findings from this project can shape future developments in art curation and management systems. The scalable model offers potential applications in museum archives, online art platforms, and educational tools. Further research could integrate additional features such as real-time analysis, multilingual support, and integration with augmented reality systems for immersive art experiences.
This AI-driven solution marks a significant step toward modernizing and democratizing access to global art collections.
This challenge is hosted with our friends at



Become an Omdena Collaborator
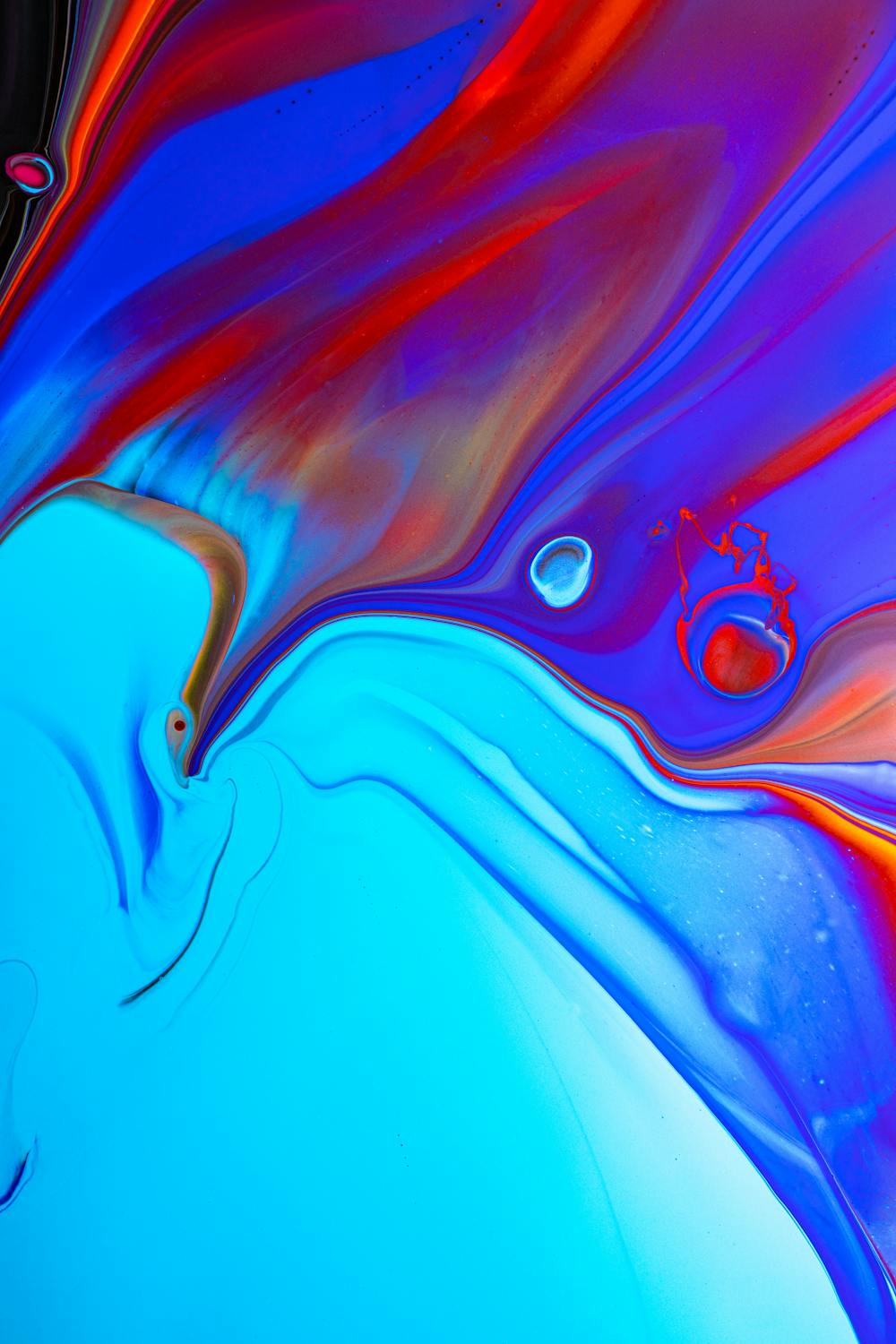