Fake Job Detection: Using NLP to Identify Fraudulent Job Postings

Background
The job market, especially for junior professionals, is riddled with fake job postings that often result in scams, loss of motivation, or even entanglement in criminal activities. Ambiguities in job titles, especially within the data science domain, exacerbate this issue, causing even experienced professionals to misunderstand job roles. Addressing these challenges is crucial to building trust and confidence in job seekers.
Objective
- Develop a machine learning model to classify job postings as safe or potentially fraudulent.
- Identify high-risk words and patterns associated with fake job postings.
- Create a word cloud visualization to highlight common terms in fraudulent job ads.
Approach
The project followed a structured methodology:
- Web Scraping: Gathered data by scraping job postings across various platforms to ensure a comprehensive dataset.
- Text Preprocessing: Cleaned and prepared textual data, removing irrelevant information and ensuring consistency.
- Feature Extraction and Model Building: Leveraged Natural Language Processing (NLP) techniques to extract features, such as word frequencies, and built machine learning models for classification.
- Model Tuning and Interpretation: Fine-tuned the models for optimal performance and interpreted results to highlight key indicators of fake job postings.
Results and Impact
- Accurate Classification: The model effectively identified fraudulent job postings, achieving high precision and recall rates.
- High-Risk Words Identified: Patterns and terms often associated with fake postings were identified, aiding job seekers in spotting red flags.
- Visualization: The word cloud visualization provided an intuitive tool to understand commonly used terms in fraudulent postings.
- Broader Impact: The project empowers job seekers by reducing their vulnerability to scams and ensuring safer navigation of the job market.
Future Implications
The insights gained from this project can influence policies aimed at regulating online job platforms. Furthermore, the models and methods developed could be expanded to other domains, such as detecting fraudulent ads in e-commerce or social media. Future research could focus on incorporating additional features like company credibility scores and expanding the dataset to include global job postings.



Become an Omdena Collaborator
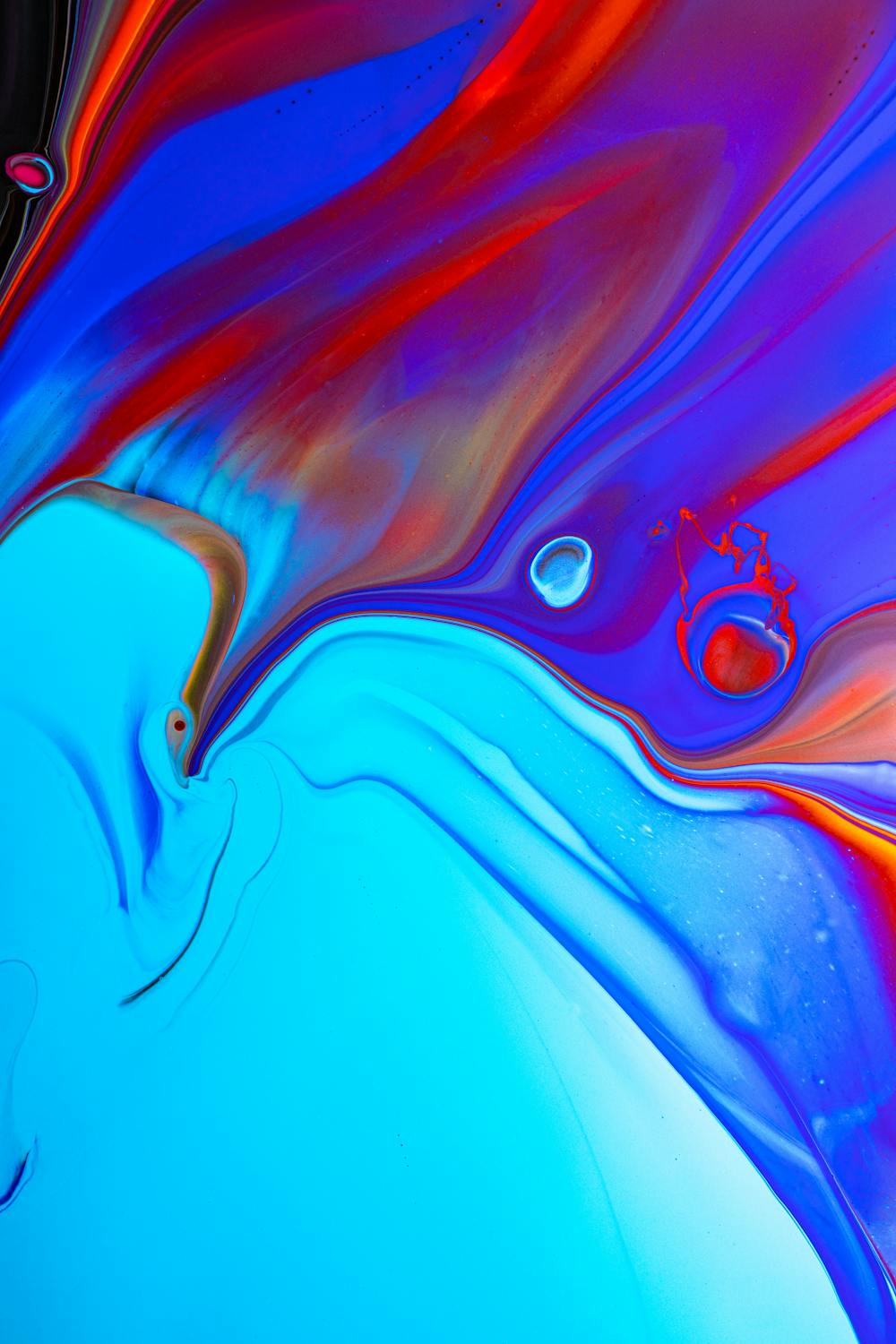