Increasing the Spatial Resolution of Satellite Images With Deep Learning

Background
The spatial resolution of Sentinel-2 satellite images, a mission by the European Space Agency (ESA), is limited to 10 meters for some bands and 20 meters for others. This resolution constraint restricts the visibility of finer details crucial for applications like land monitoring, agriculture, and forestry. To overcome this limitation, leveraging advanced deep learning techniques was proposed to enhance image resolution and utility.
Objective
The project aimed to develop a deep learning model, particularly leveraging Generative Adversarial Networks (GANs), capable of increasing the spatial resolution of Sentinel-2 satellite images by a factor of 10x. This would enable enhanced clarity and detail for the 4 RGBN bands (Red, Green, Blue, Near-Infrared) at a 16-bit depth to maximize information capture and analysis.
Approach
The problem was addressed by:
- Deep Learning Techniques: Using GANs, which generate new data samples by learning from training datasets.
- Training Dataset: High-resolution imagery paired with low-resolution Sentinel-2 images to train the model.
- Bands Used: Focus on the 4 RGBN bands at 16-bit resolution.
- Implementation Goals: Ensure the output images provide a 10x increase in resolution while maintaining data accuracy and integrity.
Results and Impact
The project successfully built a GAN model that increased the spatial resolution of Sentinel-2 satellite images by 10x. This advancement:
- Improved the clarity and usability of satellite imagery for land monitoring, agriculture, and forestry.
- Enhanced data precision by maintaining 16-bit quality for the 4 RGBN bands.
- Demonstrated the potential of GANs in addressing real-world challenges in remote sensing, contributing to efficient resource management and informed decision-making.
Future Implications
These findings highlight the transformative potential of deep learning in remote sensing, paving the way for further research in image super-resolution. The approach can inspire future policies aimed at better utilizing satellite data for environmental conservation, precision agriculture, and urban planning, while also setting a benchmark for other image enhancement challenges.
This project is hosted with our friends at



Become an Omdena Collaborator
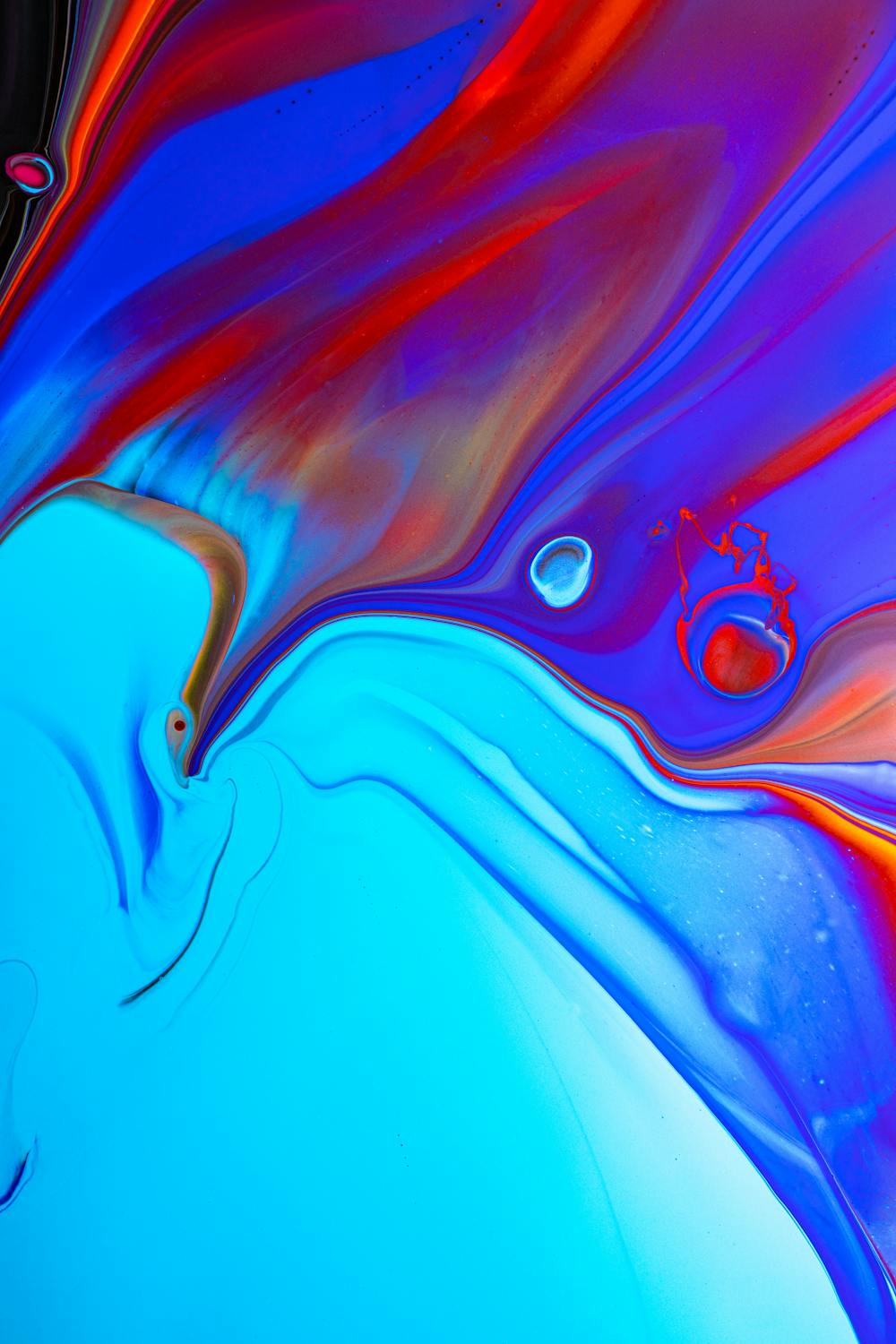