Quantifying the Impact of Forest Landscape Restoration Through Predictive Analytics

In this two-month Omdena project, 50 AI changemakers built a predictive impact analytics dashboard that can quantify the social, economic, and environmental impact of making an investment in a particular forest landscape restoration project.
The problem
Climate change could cost the world ~$792 trillion in the next 80 years. Forest landscape restoration (FLR) helps mitigate those risks e.g. mangroves absorb 70-90% of storm surge. In fact, FLR could generate $7–$30 in economic benefits for every dollar invested. Yet these co-benefits are undervalued by markets. This poses a major impediment to financing FLR, which faces an annual investment gap of around $400bn.
The mission of Trillion Tree Fund is to scale conservation finance to restore 1.2 trillion trees—which would cancel out a decade of carbon emissions. We also aim to legally represent nature on our board of trustees, in recognition of nature as our biggest social and economic partner.
The project outcomes
To encourage institutional investors to invest in FLR funds, Omdena’s collaborators built a predictive impact analytics solution that can quantify the social, economic, and environmental impact of making an investment in a particular FLR project. In addition, they developed a simple dashboard, which displays the calculation of the different impact and co-benefits expressed in USD terms.
These questions were answered:
- Investments: What inputs are needed for the calculator and what datasets need to be linked/pre-loaded? For example:
- Cost of an investment
- Tree species (e.g. mangroves protect from coastal flooding but palm trees do not; certain trees are fire resistant to wildfires but not all; some trees have greater value in increasing property values; trees have different carbon sequestration potential; some produce non-timber commodities like fruits, rubber…etc.)
- Tree maturity, Ecoregion, Land area, etc.
- Benefits: What datasets are available (and in what regions) to evaluate the potential ROI and different co-benefits of investments? Co-benefits examples:
- Reduced costs of damages or liabilities (e.g. insurance payouts for flooding, or mortgage defaults due to flooding)
- Cost of business disruptions/reduced economic productivity and supply chain issues
- Health costs (e.g. reduced cost of respiratory disease due to air filtration or cost-benefit of mental/physical health improvements e.g. reduced incidences of hypertension, obesity, diabetes).
- Increased ecotourism revenues and job creation (every ha of mangroves generates $1,000 in ecotourism revenues)
- Food security (agroforestry, fisheries enhanced by mangroves)
- Increasing property value (California’s 9 million trees increased property values by over $800m)
- Carbon sequestration
Additional outcomes:
- Adding a GIS map to the dashboard showing the cost of doing nothing, and mapping the co-benefits of an FLR project at its full restoration potential.
- Calculating how far the impact extends to e.g. 5-mile radius? 10 miles?
- Identifying who benefits from that particular investment i.e. x insurance company, x bank, x agricultural producer.
- Showing a scenario map of reduced climate risks and co-benefits at full restoration potential e.g. floodwater absorption rate or wildfire spread rate.
First Omdena Project?
Join the Omdena community to make a real-world impact and develop your career
Build a global network and get mentoring support
Earn money through paid gigs and access many more opportunities
Your Benefits
Address a significant real-world problem with your skills
Receive certificates after successful project completion
Build your Omdena project portfolio to get hired at top companies
Access paid projects, speaking gigs, writing opportunities, and much more
Requirements
Good English
A good/very good grasp in computer science and/or mathematics
Student, (aspiring) data scientist, (senior) ML engineer, data engineer, or domain expert (no need for AI expertise)
Programming experience with C/C++, C#, Java, Python, Javascript or similar
Experience in data analysis, data engineering and/or predictive analytics, and visualization
This challenge has been hosted with our friends at
Application Form



Become an Omdena Collaborator
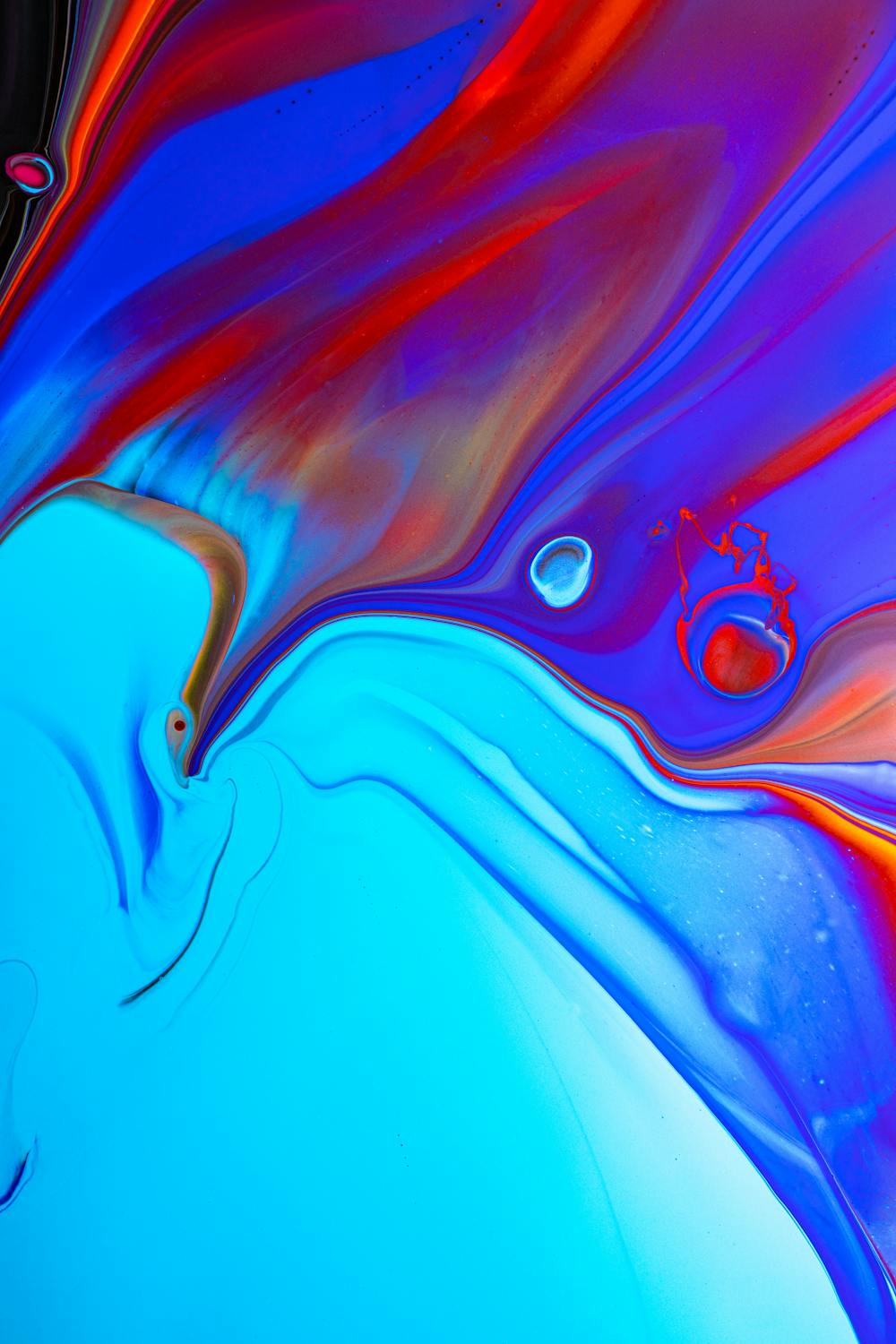