7 Learnable Personality Traits of a Happy Data Scientist
February 11, 2021

In the world of scary-looking datasets and low classification accuracy, what does it take for a data scientist to stay calm and happy?
Data Science is a fierce profession. There is constant turmoil between data engineers, data wranglers, and the continually evolving datasets. Some day you might be training endlessly on the data just to find your approach was incorrect. And then, some days, you might not even know how to proceed further. Or your work tasks depend on somebody else. You might even think of giving up on your project!
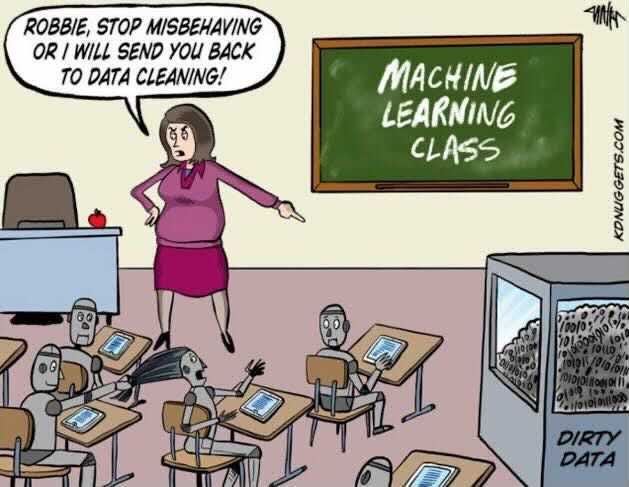
Source: Reddit
But wait, there are many important steps you can take to be more fulfilled, joyful, and happy on the job.
Why happiness counts (for a data scientist)!
After all, the benefit of happiness is obvious: it’s happiness itself — the experience of joy, contentment, or positive well-being, combined with a sense that one’s life is good, meaningful, and worthwhile.
What more do we want? The experience of happiness can feel like the ultimate aim, the ultimate experience in life, right?
While this all has truth to it, being happy also has obvious benefits for you as a data scientist. Here’s an overview of some of the secondary benefits happiness has been linked to:
- Happiness is good for our relationships. Happy people are more likely to excel at teamwork, and they have more friends (at work).
- Happiness increases cognition by enhancing attention, deepening thinking, and improving mental flexibility.
- Happy people also make more money and are more creative at work.
Data Science = Math & Psychology
Despite being an extremely technical and mathematical field, data science has a psychological aspect towards it. This entails how you practice data science and how your personality responds to different scenarios.
Data is just the world making noises.
And one has to learn to find the serenity in this noise. For a data scientist, each day can be filled with intuition, questions, doubts, confusion, or even frustration.
So here comes the master question. How can data scientists keep themselves happy while increasing the chances of their own definition of success?
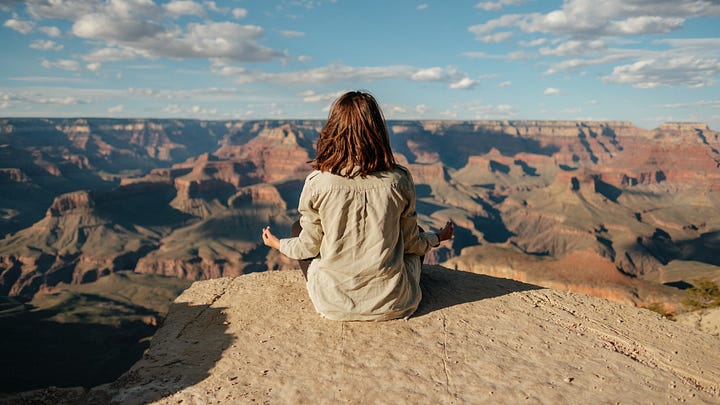
Photo by Matteo Di Iorio on Unsplash
1. Pave your own path (patiently)
“If the path before you is clear, you’re probably on someone else’s.” ― Joseph Campbell
Every individual is on a journey of their own. Some achieve success in a day, some in a month, and some in a year. Gary Vee once said, “Everything takes longer than you think.” And this is very true. In our fast-paced world, we end up wanting things too fast. We set up many expectations, and as you might already know, out of unmet expectations, we create our own headaches, anxieties, and heartaches (unnecessarily).
So, instead, as a happy data scientist, you want to formulate your own path. While your journey and ambitions might align with other people, they will never be the same. And that is just natural!
Each day new research is coming up in the field of AI. The lack of a rigid structure in this domain allows us, practitioners, to transcend into new domains. This might seem overwhelming but it also provides an opportunity to find your own path.
To put it into data science terms,
To not get lost amidst the dense jungle of “Random Forest,” you must know how to tune your body’s hyperparameters to leap across it in a single instance.
How do this? By following your individuality and questioning the common path. Try out things and learn from many experiences. The more you do this, the more you get to know yourself and carve out your own path in the world of look-alike data scientists. Be unique, because you are!
2. Realize you’re here for the long run
“Enthusiasm is common. Endurance is rare.” ― Angela Duckworth
Next to being patient, resilience is at the core of becoming a good data scientist. You need to wait for enough data to be collected, appropriate features, classes to be balanced, and then fine-tune your Neural Network on your dataset. Data science takes time, and it’s important to not give up in the middle of it, just because you bump into a few obstacles.
A good way to become more resilient is to keep the big picture in your mind.
Whenever your code hits a roadblock you cannot figure out, remind yourself why you started doing this project in the first place. Why am I in it?
A good way to make sure of this is to have a rough idea written down somewhere where you outline your project’s entire pipeline from start to finish. Don’t be discouraged if your model is not training or your classes are imbalanced. The chances are that others in the community have faced the same problem and have shared their helpful tips on online forums for you.
Instead of dumping it, try to approach it from a different angle. Online resources, articles, and GitHub repositories are some good places to get help from. It is also vital to allocate an appropriate amount of time to every leg of the project. For example, modeling should not take the same amount of time as data preprocessing.
3. Engage in meaningful work
“Turn your passion into cause. For that’s when you discover the true essence of it.” — Anonymous
Data science is often being used to help businesses drive efficiencies and ultimately generate more revenue. But that is not all! In reality, data science is building the future of our world. To be truly happy at anything, you must see a bigger purpose in it. Choose a project that inspires or motivates you to work rigorously on it. Here are a few of those projects.
Emotional commitment is the key to achieving mastery.
The best part about this interdisciplinary field is that you can create your own project. Maybe you want to use neural nets to classify your music collection. Or to predict your favorite stock price. Or even make beautiful realistic art. Find a project you care about and you can ignore other ventures.
Struggling with a project you care about will teach you far more than working on something you are not passionate about.
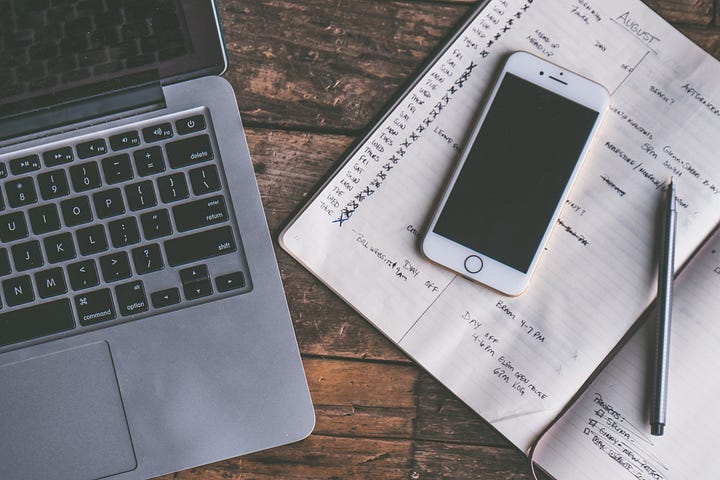
Photo by on Unsplash
4. Create a learning check-list
“Absorb what is useful, reject what is useless, add what is specifically your own.” — Bruce Lee
There will come a time in your journey when you will feel as if you’re stagnating and not learning or doing enough. Instead of falling down the guilt-ridden road of comparing yourself to others, choose to make a learning checklist for yourself instead. It could be for a week or a month (or even a single day!). The purpose is not to bound yourself to some arbitrarily set goals but to emotionally and mentally reward yourself when you finish them. Start with small, very achievable goals. Once you finish them, make the next checklist for the same frequency. The key is to keep going at a non-zero velocity. Your mind will intrinsically reward you for achieving your goals, don’t ignore that small pat on the back; embrace it. Once you start finding it easy to have a checklist for yourself, you will push yourself to learn more.
And if you must compare yourself to others, instead of comparing your achievements against theirs, compare your checklists with theirs. Chances are the people you look up to are doing well because they adhere to a proper timeline on their learning activities and projects.
The secret mantra is universally simple to remember: you should keep moving forward at a steadily increasing non-zero velocity (if you want a challenge, add some acceleration to it).
5. Break down a problem into chunks
Data is the heart and soul of a data science problem, and engineers work endlessly to tune some signals out of the noisy raw data. It will often be the case that you are working on some clean features and attributes yet still not getting any output. The case is that you are examining the problem at a macro scale, whereas the answer is disguised in the form of its micro characteristics. A simple example to explain my point is the training of a model to detect faces, where you start from a bottom-up approach. You begin by detecting contours, skin-tone, or the presence/absence of a single-pixel! Finally, build your way up to detecting complex facial features like eyes, nose, or mouth. Similarly, being detail-oriented in your working environment and breaking the problem into chunks differentiates your task into simplex-achievable feats.
And with the completion of each micro task, you receive micro rewards as well!
Solving each mini-task is synonymous with climbing the ladder of success. You reward yourself at each stage with increased accuracy and confidence in your models. This way, your hard work is preserved and not lost if any one of your sub-problem fails.
6. Help others (and work in a team)
“Individual commitment to a group effort — that is what makes a team work, a company work, a society work, a civilization work.”— Vince Lombardi
Since its inception, Knowledge-based learning has evolved into a wide variety of categories. Almost every problem today requires a variety of expertise in different domains to address it. You might require a Deep Learning expert, an NLP engineer, or a GAN specialist. So it is essential that you have the spirit of teamwork in you. It is necessary to be able to communicate your requirements for a particular task to the problem. It is often the case in Data Science that one discovers a person through their data. Therefore it is important to comprehend the problems faced by your team.
Take a break from your grilling session of programming and talk to people around you. Ask their views on your work and reciprocate the same. Don’t be afraid to take assistance from your team.
Start interacting with people actively participating in the AI community. Be polite, ask them about their journey, document their mistakes, and understand their determination source. We must remember that asking for help and helping are two aspects of the same coin that make life worthwhile.
Photo by heylagostechie on Unsplash
7. Read more (but not too much)
“Reading one research paper a day keeps the imposter syndrome away.” — Anonymous
Staying familiar with the latest research is crucially important for researchers and practitioners. Even if your work does not involve delving deep into multivariable calculus to modify and develop the next state-of-the-art optimizer that beats all the others, you will find that being on good terms with the latest papers in the field will help you tremendously in your projects.
Before you skip this point and move to the next one, let us assure you it is really not that hard. Reading one paper takes no more than 30 minutes (once you get started with it). The important aspect to remember is that you are likely to give up on this habit if you read state-of-the-art papers that are too complicated for you to understand in the beginning. Find something you are genuinely interested in. No one will ask you to justify the papers you read; it is entirely up to you and your interest. Okay, so now you have your decided field. How do you find papers? There are a plethora of resources on the internet and before you train yourself in using all of them, start with places like paperswithcode.com, Google scholar, and IEEE and Springer journals.
- Pick 2 papers out of the ones you see.
- Don’t spend more than 10 minutes picking the papers.
- Don’t read the whole paper when you choose it; just glimpse over the abstract, nothing more.
- When it’s time to start reading the two papers (both papers NOT on the same day), you will need a pen and paper, preferably. Watch this video where Dr. Andrew Ng shares his advice on how to go about reading a Machine Learning paper.
- Only when you are very comfortable is when you increase by 1 paper a week and then continue for at least a month. Continuing this habit will make you very comfortable reading new papers and participating in paper reading sessions with others.
Bonus tip: Don´t avoid mistakes; avoid repeating mistakes
“One of the basic rules of the universe is that nothing is perfect. Perfection simply doesn’t exist. Without imperfection, neither you nor I would exist”― Stephen Hawking
You are perfect in your imperfection. We are all. The problem is if we stop growing. And the only way to grow is to make mistakes and forgive yourself to move on.

Source: Canva
Truth be told, every mistake is a success in itself. Every data scientist begins their career with a free ride on the sine wave of AI. The crests and troughs tend to eventually wear down a person, and thus to avoid getting lost among the intricacies, a mistake comes to the rescue. The curiosity to mend your faults and failures motivates you to go deep into the depths of the subject. Many veterans have always pointed out that understanding their mistakes and trying to solve errors is the only thing that can help you grow and learn. Rectifying the wrong choice of models and errors forms a dictionary of success in which you are constantly adding your precious entries.
Develop the resolution to challenge yourself with mistakes and the diligence to understand your own responsibility towards your goals and codes.
Conclusion
What does it mean to be a Happy Data Scientist? Do your models train better? Your dataset is clean as a whistle? While there are a few common patterns, the exact sources of happiness will be different for each one of us.
But what’s not different is the enthusiasm we all share for this field. The excitement in learning about a new type of model. The first time your model’s test accuracy is above 95% or when you deploy your first model over a Flask API and host it online.
As long as you are genuinely interested in the field and possess the will to continue learning and embracing the magic it has to offer, you will find a way to be happy.
This article has been written by Mohit Sharma, Ramansh Sharma, and Guneet Kohli through Omdena’s blog Writers’ Academy.
A good way to practice these points is to do it in a collaborative setting with other data scientists. Check out some of Omdena’s AI projects and apply to them to participate in a two month long challenge to make a positive impact as a real-world data scientist.



